mirror of
https://github.com/xiph/opus.git
synced 2025-05-14 23:48:28 +00:00
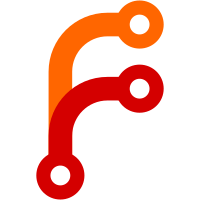
Retraining a new model so that we have a matching .pth file. Also, discarding more data on init to get better randomization.
192 lines
6 KiB
C
192 lines
6 KiB
C
/* Copyright (c) 2023 Amazon */
|
|
/*
|
|
Redistribution and use in source and binary forms, with or without
|
|
modification, are permitted provided that the following conditions
|
|
are met:
|
|
|
|
- Redistributions of source code must retain the above copyright
|
|
notice, this list of conditions and the following disclaimer.
|
|
|
|
- Redistributions in binary form must reproduce the above copyright
|
|
notice, this list of conditions and the following disclaimer in the
|
|
documentation and/or other materials provided with the distribution.
|
|
|
|
THIS SOFTWARE IS PROVIDED BY THE COPYRIGHT HOLDERS AND CONTRIBUTORS
|
|
``AS IS'' AND ANY EXPRESS OR IMPLIED WARRANTIES, INCLUDING, BUT NOT
|
|
LIMITED TO, THE IMPLIED WARRANTIES OF MERCHANTABILITY AND FITNESS FOR
|
|
A PARTICULAR PURPOSE ARE DISCLAIMED. IN NO EVENT SHALL THE FOUNDATION OR
|
|
CONTRIBUTORS BE LIABLE FOR ANY DIRECT, INDIRECT, INCIDENTAL, SPECIAL,
|
|
EXEMPLARY, OR CONSEQUENTIAL DAMAGES (INCLUDING, BUT NOT LIMITED TO,
|
|
PROCUREMENT OF SUBSTITUTE GOODS OR SERVICES; LOSS OF USE, DATA, OR
|
|
PROFITS; OR BUSINESS INTERRUPTION) HOWEVER CAUSED AND ON ANY THEORY OF
|
|
LIABILITY, WHETHER IN CONTRACT, STRICT LIABILITY, OR TORT (INCLUDING
|
|
NEGLIGENCE OR OTHERWISE) ARISING IN ANY WAY OUT OF THE USE OF THIS
|
|
SOFTWARE, EVEN IF ADVISED OF THE POSSIBILITY OF SUCH DAMAGE.
|
|
*/
|
|
|
|
/* This packet loss simulator can be used independently of the Opus codebase.
|
|
To do that, you need to compile the following files:
|
|
dnn/lossgen.c
|
|
dnn/lossgen_data.c
|
|
|
|
with the following files needed as #include
|
|
dnn/lossgen_data.h
|
|
dnn/lossgen.h
|
|
dnn/nnet_arch.h
|
|
dnn/nnet.h
|
|
dnn/parse_lpcnet_weights.c (included despite being a C file)
|
|
dnn/vec_avx.h
|
|
dnn/vec.h
|
|
celt/os_support.h
|
|
celt/arch.h
|
|
celt/x86/x86_arch_macros.h
|
|
include/opus_defines.h
|
|
include/opus_types.h
|
|
|
|
Additionally, the code in dnn/lossgen_demo.c can be used to generate losses from
|
|
the command line.
|
|
*/
|
|
|
|
#ifdef HAVE_CONFIG_H
|
|
#include "config.h"
|
|
#endif
|
|
|
|
#include "arch.h"
|
|
|
|
#include <math.h>
|
|
#include "lossgen.h"
|
|
#include "os_support.h"
|
|
#include "nnet.h"
|
|
#include "assert.h"
|
|
|
|
/* Disable RTCD for this. */
|
|
#define RTCD_ARCH c
|
|
|
|
/* Override assert to avoid undefined/redefined symbols. */
|
|
#undef celt_assert
|
|
#define celt_assert assert
|
|
|
|
/* Directly include the C files we need since the symbols won't be exposed if we link in a shared object. */
|
|
#include "parse_lpcnet_weights.c"
|
|
#include "nnet_arch.h"
|
|
|
|
#undef compute_linear
|
|
#undef compute_activation
|
|
|
|
/* Force the C version since the SIMD versions may be hidden. */
|
|
#define compute_linear(linear, out, in, arch) ((void)(arch),compute_linear_c(linear, out, in))
|
|
#define compute_activation(output, input, N, activation, arch) ((void)(arch),compute_activation_c(output, input, N, activation))
|
|
|
|
#define MAX_RNN_NEURONS_ALL IMAX(LOSSGEN_GRU1_STATE_SIZE, LOSSGEN_GRU2_STATE_SIZE)
|
|
|
|
/* These two functions are copied from nnet.c to make sure we don't have linking issues. */
|
|
void compute_generic_gru_lossgen(const LinearLayer *input_weights, const LinearLayer *recurrent_weights, float *state, const float *in, int arch)
|
|
{
|
|
int i;
|
|
int N;
|
|
float zrh[3*MAX_RNN_NEURONS_ALL];
|
|
float recur[3*MAX_RNN_NEURONS_ALL];
|
|
float *z;
|
|
float *r;
|
|
float *h;
|
|
celt_assert(3*recurrent_weights->nb_inputs == recurrent_weights->nb_outputs);
|
|
celt_assert(input_weights->nb_outputs == recurrent_weights->nb_outputs);
|
|
N = recurrent_weights->nb_inputs;
|
|
z = zrh;
|
|
r = &zrh[N];
|
|
h = &zrh[2*N];
|
|
celt_assert(recurrent_weights->nb_outputs <= 3*MAX_RNN_NEURONS_ALL);
|
|
celt_assert(in != state);
|
|
compute_linear(input_weights, zrh, in, arch);
|
|
compute_linear(recurrent_weights, recur, state, arch);
|
|
for (i=0;i<2*N;i++)
|
|
zrh[i] += recur[i];
|
|
compute_activation(zrh, zrh, 2*N, ACTIVATION_SIGMOID, arch);
|
|
for (i=0;i<N;i++)
|
|
h[i] += recur[2*N+i]*r[i];
|
|
compute_activation(h, h, N, ACTIVATION_TANH, arch);
|
|
for (i=0;i<N;i++)
|
|
h[i] = z[i]*state[i] + (1-z[i])*h[i];
|
|
for (i=0;i<N;i++)
|
|
state[i] = h[i];
|
|
}
|
|
|
|
|
|
void compute_generic_dense_lossgen(const LinearLayer *layer, float *output, const float *input, int activation, int arch)
|
|
{
|
|
compute_linear(layer, output, input, arch);
|
|
compute_activation(output, output, layer->nb_outputs, activation, arch);
|
|
}
|
|
|
|
|
|
static int sample_loss_impl(
|
|
LossGenState *st,
|
|
float percent_loss)
|
|
{
|
|
float input[2];
|
|
float tmp[LOSSGEN_DENSE_IN_OUT_SIZE];
|
|
float out;
|
|
int loss;
|
|
LossGen *model = &st->model;
|
|
input[0] = st->last_loss;
|
|
input[1] = percent_loss;
|
|
compute_generic_dense_lossgen(&model->lossgen_dense_in, tmp, input, ACTIVATION_TANH, 0);
|
|
compute_generic_gru_lossgen(&model->lossgen_gru1_input, &model->lossgen_gru1_recurrent, st->gru1_state, tmp, 0);
|
|
compute_generic_gru_lossgen(&model->lossgen_gru2_input, &model->lossgen_gru2_recurrent, st->gru2_state, st->gru1_state, 0);
|
|
compute_generic_dense_lossgen(&model->lossgen_dense_out, &out, st->gru2_state, ACTIVATION_SIGMOID, 0);
|
|
loss = (float)rand()/RAND_MAX < out;
|
|
st->last_loss = loss;
|
|
return loss;
|
|
}
|
|
|
|
int sample_loss(
|
|
LossGenState *st,
|
|
float percent_loss)
|
|
{
|
|
/* Due to GRU being initialized with zeros, the first packets aren't quite random,
|
|
so we skip them. */
|
|
if (!st->used) {
|
|
int i;
|
|
for (i=0;i<1000;i++) sample_loss_impl(st, percent_loss);
|
|
st->used = 1;
|
|
}
|
|
return sample_loss_impl(st, percent_loss);
|
|
}
|
|
|
|
void lossgen_init(LossGenState *st)
|
|
{
|
|
int ret;
|
|
OPUS_CLEAR(st, 1);
|
|
ret = init_lossgen(&st->model, lossgen_arrays);
|
|
celt_assert(ret == 0);
|
|
(void)ret;
|
|
}
|
|
|
|
int lossgen_load_model(LossGenState *st, const void *data, int len) {
|
|
WeightArray *list;
|
|
int ret;
|
|
parse_weights(&list, data, len);
|
|
ret = init_lossgen(&st->model, list);
|
|
opus_free(list);
|
|
if (ret == 0) return 0;
|
|
else return -1;
|
|
}
|
|
|
|
#if 0
|
|
#include <stdio.h>
|
|
int main(int argc, char **argv) {
|
|
int i, N;
|
|
float p;
|
|
LossGenState st;
|
|
if (argc!=3) {
|
|
fprintf(stderr, "usage: lossgen <percentage> <length>\n");
|
|
return 1;
|
|
}
|
|
lossgen_init(&st);
|
|
p = atof(argv[1]);
|
|
N = atoi(argv[2]);
|
|
for (i=0;i<N;i++) {
|
|
printf("%d\n", sample_loss(&st, p));
|
|
}
|
|
}
|
|
#endif
|